如何使用Python和OpenCV实现对象检测任务的数据扩充过程?
def colorjitter(img, cj_type="b"):
'''
### Different Color Jitter ###
img: image
cj_type: {b: brightness, s: saturation, c: constast}
'''
if cj_type == "b":
# value = random.randint(-50, 50)
value = np.random.choice(np.array([-50, -40, -30, 30, 40, 50]))
hsv = cv2.cvtColor(img, cv2.COLOR_BGR2HSV)
h, s, v = cv2.split(hsv)
if value >= 0:
lim = 255 - value
v[v > lim] = 255
v[v <= lim] += value
else:
lim = np.absolute(value)
v[v < lim] = 0
v[v >= lim] -= np.absolute(value)
final_hsv = cv2.merge((h, s, v))
img = cv2.cvtColor(final_hsv, cv2.COLOR_HSV2BGR)
return img
elif cj_type == "s":
# value = random.randint(-50, 50)
value = np.random.choice(np.array([-50, -40, -30, 30, 40, 50]))
hsv = cv2.cvtColor(img, cv2.COLOR_BGR2HSV)
h, s, v = cv2.split(hsv)
if value >= 0:
lim = 255 - value
s[s > lim] = 255
s[s <= lim] += value
else:
lim = np.absolute(value)
s[s < lim] = 0
s[s >= lim] -= np.absolute(value)
final_hsv = cv2.merge((h, s, v))
img = cv2.cvtColor(final_hsv, cv2.COLOR_HSV2BGR)
return img
elif cj_type == "c":
brightness = 10
contrast = random.randint(40, 100)
dummy = np.int16(img)
dummy = dummy * (contrast/127+1) - contrast + brightness
dummy = np.clip(dummy, 0, 255)
img = np.uint8(dummy)
return img
添加噪声通常,噪声被认为是图像中不可预料的因素,然而,有几种类型的噪声(如高斯噪声、椒盐噪声)可以用于数据扩充,在深度学习中,添加噪声是一种非常简单而有益的数据扩充方法。在下面的例子中,为了增强数据,将高斯噪声和椒盐噪声添加到原始图像中。
对于那些无法识别高斯噪声和椒盐噪声区别的人,高斯噪声的取值范围取决于配置,从0到255,因此,在RGB图像中,高斯噪声像素可以是任何颜色。相反,椒盐噪声像素只能有两个值:0或255,分别为黑色(椒)或白色(盐)。def noisy(img, noise_type="gauss"):
'''
### Adding Noise ###
img: image
cj_type: {gauss: gaussian, sp: salt & pepper}
'''
if noise_type == "gauss":
image=img.copy()
mean=0
st=0.7
gauss = np.random.normal(mean,st,image.shape)
gauss = gauss.astype('uint8')
image = cv2.add(image,gauss)
return image
elif noise_type == "sp":
image=img.copy()
prob = 0.05
if len(image.shape) == 2:
black = 0
white = 255
else:
colorspace = image.shape[2]
if colorspace == 3: # RGB
black = np.array([0, 0, 0], dtype='uint8')
white = np.array([255, 255, 255], dtype='uint8')
else: # RGBA
black = np.array([0, 0, 0, 255], dtype='uint8')
white = np.array([255, 255, 255, 255], dtype='uint8')
probs = np.random.random(image.shape[:2])
image[probs < (prob / 2)] = black
image[probs > 1 - (prob / 2)] = white
return image
过滤本文介绍的最后一个数据扩充过程是过滤。与添加噪声类似,过滤也很简单,易于实现。在实现中使用的三种滤波类型包括模糊(均值)、高斯和中值。
def filters(img, f_type = "blur"):
'''
### Filtering ###
img: image
f_type: {blur: blur, gaussian: gaussian, median: median}
'''
if f_type == "blur":
image=img.copy()
fsize = 9
return cv2.blur(image,(fsize,fsize))
elif f_type == "gaussian":
image=img.copy()
fsize = 9
return cv2.GaussianBlur(image, (fsize, fsize), 0)
elif f_type == "median":
image=img.copy()
fsize = 9
return cv2.medianBlur(image, fsize)
总结
在这篇文章中,主要向大家介绍了一个关于对象检测任务中数据扩充实现的教程。你们可以在这里找到完整实现。https://github.com/tranleanh/data-augmentation
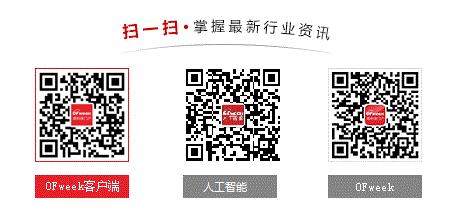
最新活动更多
-
3月27日立即报名>> 【工程师系列】汽车电子技术在线大会
-
4月30日立即下载>> 【村田汽车】汽车E/E架构革新中,新智能座舱挑战的解决方案
-
5月15-17日立即预约>> 【线下巡回】2025年STM32峰会
-
即日-5.15立即报名>>> 【在线会议】安森美Hyperlux™ ID系列引领iToF技术革新
-
5月15日立即下载>> 【白皮书】精确和高效地表征3000V/20A功率器件应用指南
-
5月16日立即参评 >> 【评选启动】维科杯·OFweek 2025(第十届)人工智能行业年度评选
发表评论
请输入评论内容...
请输入评论/评论长度6~500个字
暂无评论
暂无评论